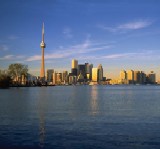 |
MINISYMPOSIA |
Numerical
differential equations and their applications, II
organized by
Anita Layton, Duke University
Wayne Hayes (Imperial College London)
Ned Nedialkov (McMaster University)
|
This
minisymposium, to be held in honor of Professor Kenneth Jackson's 60th
birthday, will survey novel discretization techniques in numerical differential
equations that address the computational challenges posed by high index,
low regularity, long-time integration, etc. Topics include mathematical
finance, simulations of the solar system, computational fluid dynamics,
and medical imaging.
Meng
Han,
University of Toronto
|
A
novel approximation to loss probabilities of credit portfolios
Credit risk analysis and management at the portfolio level are challenging
problems for financial institutions due to their portfolios' large
size, heterogeneity and complex correlation structure. The conditional-independence
framework is widely used to calculate loss probabilities of credit
portfolios. The existing computational approaches within this framework
fall into two categories: (1) simulation-based approximations and
(2) asymptotic approximations. The simulation-based approximations
often involve a two-level Monte Carlo method, which is extremely
time-consuming, while the asymptotic approximations, which are typically
based on the Law of Large Number (LLN), are not accurate enough
for tail probabilities, especially for heterogeneous portfolios.
We give a more accurate asymptotic approximation based on the Central
Limit Theorem (CLT), and we discuss when it can be applied. To further
increase accuracy, we also propose a hybrid approximation, which
combines the simulation-based approximation and the asymptotic approximation.
We test our approximations with some artificial and real portfolios.
Numerical examples show that, for a similar computational cost,
the CLT approximation is more accurate than the LLN approximation
for both homogeneous and heterogeneous portfolios, while the hybrid
approximation is even more accurate than the CLT approximation.
Moreover, the hybrid approximation significantly reduces the computing
time for comparable accuracy compared to simulation-based approximations.
|
Nargol
Rezvani University of Toronto
|
A
polyenergetic model and iterative reconstruction framework for X-ray
computerized tomography (CT)
While most modern x-ray CT scanners rely on the well-known filtered
back-projection (FBP) algorithm, the corresponding reconstructions
can be corrupted by beam-hardening artifacts. These artifacts arise
from the unrealistic physical assumption of monoenergetic x-ray
beams. To compensate, we discretize an alternative model directly
that accounts for differential absorption of polyenergetic x-ray
photons. We present numerical reconstructions based on the associated
nonlinear discrete formulation incorporating various iterative optimization
frameworks. This is joint work with D. Aruliah and K. Jackson.
|
Dongwoon
Lee
University of Toronto
|
Geometric
reconstruction and physical simulation of human skeletal muscle
Data from human cadavers enable us to obtain in-depth understanding
and accurate parameter estimation of human skeletal muscle. However,
as most muscles have non-homogeneous architecture, it is not straightforward
to determine their parameters. Thus, we propose a geometric approach
in which volumes of fiber bundles are individually approximated
as parametric solids and aggregated to determine the architecture
and geometry of human skeletal muscle. Lumped-parameter models are
commonly used in many computational studies of human skeletal muscle.
However, the simplified representation of architectural parameters
inherent in these models often limits their ability to determine
morphology and mechanical functions of complex muscles. Therefore,
we are developing micro-mechanical muscle models to incorporate
variable architectural parameters and non-uniform fiber arrangements,
enabling us to predict contractile behaviors of complex muscles.
|
Duy
Minh Dang
University of Toronto |
An
efficient GPU-based parallel algorithm for pricing multi-asset American
options
We develop highly-efficient parallel Partial Differential Equation
(PDE) based pricing methods on Graphics Processing Units (GPUs)
for multi-asset American options. Our pricing approach is built
upon a combination of a discrete penalty approach for the linear
complementarity problem arising due to the free boundary and a GPU-based
parallel Alternating Direction Implicit Approximate Factorization
technique with finite differences on uniform grids for the solution
of the linear algebraic system arising from each penalty iteration.
A timestep size selector implemented efficiently on GPUs is used
to further increase the efficiency of the methods. We demonstrate
the efficiency and accuracy of the parallel numerical methods by
pricing American options written on three assets. |
|